Proximity sensors provide an effective, accurate and cheap alternative for measuring dam pedigree of lambs in Australian sheep flocks
Beth Paganoni, DPIRD Bunbury, WA; Andrew van Burgel, DPIRD Albany, WA; Claire Macleay, DPIRD Bunbury, WA; Peter Rowe, Fitprofits Perth, WA; Vicki Scanlan and Andrew Thompson, Murdoch University Perth, WA
Author correspondence: beth.paganoni@dpird.wa.gov.au
Summary
Breeding sheep for improved production traits can be achieved more rapidly if we know their parentage. Parentage of lambs is expensive and laborious to measure so we used proximity sensors to count the interactions between ewes (dams) and lambs to determine their parentage. The sensors provided a rapid result that was 97% accurate compared to DNA (Paganoni et al. 2021). In this article, we report briefly on these results and on the economics of different methods of measuring dam pedigree on-farm.
Background
Determining dam pedigree has benefits for both commercial sheep producers and ram breeders. Ram breeders recording both sire and dam pedigree are making the most rapid genetic gain across a range of traits, especially for Merinos. More rapid genetic gain is possible because determining dam pedigree improves the accuracy of breeding values and enables the adjustment for dam effects, such as her joining weight, age and birth and rear type. Most important, dam pedigree enables the generation of breeding values for reproduction traits, including the number of lambs weaned. Sires with accurate breeding values for number of lambs weaned are scarce on our national genetics database (Sheep Genetics Australia), and we estimate that less than 10% of Merino rams are sold with breeding values for reproduction. This limits the genetic gain in number of lambs weaned from Merinos for all sheep producers. Knowledge of dam pedigree also enables the culling of non-performers and within generation improvements in weaning rates (Lee et al. 2009), which is a valuable tool for ram breeders and commercial producers. Despite these potential gains in productivity, determining dam pedigree is not common because current methods are resource heavy.
Current methods for establishing dam pedigree include the conventional tagging and recording of lambs to dams during lambing (lambing rounds), matching dams to their lambs in yards pre-marking, setting up Pedigree Matchmaker (Richards et al. 2007) and measuring DNA parentage from blood samples (Dodds et al. 2005). DNA parentage is the most accurate method to determine pedigree and is therefore considered the gold standard, but it is also more costly and not immune from sampling errors. The sheep industry would benefit from developing alternative, cheaper methods. Proximity sensors, for example, were used to establish dam pedigree with 100% accuracy after 15 minutes using a small flock of 23 twin-born lambs and their dams (Sohi et al. 2017). Given that dams and their newborn lambs do not interact differently with other ewes in large or small flocks (Lockwood et al. 2018), it is likely that using proximity sensors on larger commercial flocks would be equally successful at determining dam pedigree. The first aim of this study was to ascertain whether proximity sensors could accurately and rapidly determine dam pedigree under larger commercial-scale flocks, irrespective of variations in age, birth type or flock sizes (Paganoni et al. 2021). The second aim was to determine whether this technology is cheaper than current methods.
Sites
During 2016 and 2017, proximity sensors were fitted to lambs (n=6747) and their dams across 32 flocks from 18 properties. Fifteen of the properties (27 flocks) were Merino, while the remaining three properties were Dorper (1 flock) or composite maternals (4 flocks). One third of lambs (n=2215) were from research flocks in Western Australia and the remaining lambs (n=4532) were from commercial ram-breeding properties.
Dam pedigree
Dam pedigree was collected on-farm by one or more of four methods: (i) Pedigree Matchmaker (PMM); (ii) Mothering up in pens; (iii) Mothering up at birth; and (iv) DNA analysis. For the properties that performed mothering up at birth, twice-daily lambing rounds were performed. For the properties that performed mothering up in pens, suckling determined a successful match. For the DNA analysis, blood cards were collected at marking, weaning or early post-weaning, and submitted to Sheep Genetics Australia for analysis. DNA samples were collected using various methods across properties, including tail blood at marking, direct blood sampling from the jugular, or by taking a blood or tissue sample from the ear. Five properties failed to collect or provide any on-farm pedigree data, affecting about one fifth of lambs measured (n=1475). These lambs could be used for analysis of sensor success (achieving a ewe-lamb match) but not analysis of sensor accuracy (achieving a correct ewe-lamb match).
Sensors
A minimum period of two days of interaction data was recorded for each sheep at most sites. Interactions between ewes and lambs were counted via Bluetooth at 30hz over a 1-15 metre range using ActiGraph GT3X sensors (v4.4.0 Pensacola Florida USA). Ewes were fitted with beacons (emitting a signal) and lambs were fitted with receivers (receiving signals).
The sensors were attached to collars with electronic identification numbers and were paired with the matching electronic identification number on the animal ear tag, using an XRS2 TruTest stick reader (Datamars, Banyo, Queensland, Australia). Pairing of tags to collars was done at the time of collar fitting and at removal. Less than 1% of all sensors (148 of the 15 373 sensors fitted) failed or were lost at removal. Ewes and lambs were managed under normal farm and grazing conditions whilst wearing the collars.
Sensor success and accuracy
The ewe with the most interactions with each lamb was identified as the first dam. The ewe with the second most interactions for each lamb was identified as the second dam. A minimum ratio of interactions between the first and second dam was used to determine a successful match of lamb to ewe. The success of the sensors at matching ewes to lambs was categorised as high or low: the minimum ratio that achieved 90% accuracy between the sensor and the DNA or mothering up was categorised as a ’high confidence’ or successful match. The interaction ratios that achieved <90% accuracy between the sensor and DNA were categorised as ‘low confidence’ or unsuccessful matches. To calculate the accuracy of the sensors, the match achieved by the sensor was compared to the match achieved by DNA or mothering up, as these two methods are considered more accurate than Pedigree Matchmaker. On-farm pedigree results were collected by mothering up at birth and/or DNA analysis for 20 flocks, with a total of 3859 lambs.
Economic analysis
The ActiGraph devices were designed for human studies on movement and sleep analysis, and it was not envisaged they would be used for the purpose of determining pedigree commercially. However, in 2018, a commercial proximity sensor was released for the purpose of determining dam pedigree in sheep using Bluetooth. The commercial sensor uses a protocol like this project, so we used it as a case study to determine the economic cost of using proximity sensors to determine dam pedigree. We analysed the net present value (NPV) of using the sensors over 10 years, as the assumed life of the system. An NPV allocates the capital cost spent up front across the life of the investment. The other benefit of using an NPV calculation was it takes the time value of money into account. To compare against the cost of the commercial sensors, we did an additional NPV analysis on data provided by six properties from WA that used one of four pedigree methods: DNA analysis; yard mothering up; birth mothering up; or Pedigree Matchmaker.
We assumed there were 400 ewes and 400 lambs that needed dam pedigree to be established, and that two days prior to marking or weaning, the lambs and ewes were mustered into the yards and separated to have the collars with sensors fitted. This took, one person one hour to muster and another hour to separate the mob in the yard. We budgeted for three people in the yards to fit the collars with sensors on both the ewes and the lambs, one to set up the system, record the tag/electronic identification (eID) number and link it to the sensor, and two people to work the sheep. Four hours was anticipated to tag both the lambs and ewes, and 60 minutes was allocated for collar removal from lambs, when done at marking or weaning. An extra 80 minutes was estimated for removal of the collars from the ewes.
Commercial sensors with collars cost $12.50 each and are needed for each animal (lamb and ewe). The sensors can be used over multiple mobs within the same year by reallocating them to another sheep. The system requires an Android device, costed at $600, that programs the sensor, records sheep eIDs and downloads the data. Ongoing costs are low, requiring battery replacement every three years. Replacement batteries cost $1 each and 100 sensors can be changed in an hour. Analysing the data costs $2.50 per animal per year. The assumption used to determine the cost per lamb included the cost of capital at 6% (long term return cash return from Planfarm Bankwest Benchmarks of 4% plus assumed 2% increase in land value). Wages were costed at $87 000 per year or $38 per hour plus on costs. Labour is assumed to be an experienced farm hand, and wage inflation was set at 2%. Technology inflation was set at 0%. Overall operating costs of the system were 25 hours of labour each year or $1200 in wages. The cost to analyse the data was $2000. The cost of replacing the batteries every three years was approximately $600.
Results and discussion
Proximity sensors were observed to be robust compared to other methods of dam pedigree across a range of conditions experienced on commercial properties across southern Australia. The average accuracy of the sensors was 98% compared to DNA and/or mothering up at birth as the farm pedigree method. The average success rate of the sensors in identifying a lamb to ewe was 94%, which was the same as DNA (94%), significantly better than pedigree matchmaker (90%; p<0.1), but poorer than mothering up at birth (98%; p<0.05). We therefore concluded that proximity sensors can determine dam pedigree as well as DNA (Paganoni et al. 2021).
Proximity sensors matched 94% of lambs to ewes, indicating that the sensors were successful at achieving a dam-lamb match. The lambs with an interaction ratio above two were categorised as ‘high confidence’ matches and was achieved for 98% of lambs matched. The accuracy of these high confidence matches was 97%. Lambs with interaction ratios less than two were categorised as ‘low confidence’ matches. Only 2.5% of all lambs matched had interaction ratios less than two, so there was little sensitivity to the choice of criteria we used to determine sensor success (Paganoni et al. 2021).
A confident dam match was achieved after 20 hours of wearing the proximity sensors. Across all 32 flocks, the success of proximity sensors in matching a lamb to a ewe was 90% after seven hours of wear time, increasing to 95% after 11 hours and 99% after 20 hours, indicating rapid re-establishment of the ewe-lamb bond following mustering and fitting of the sensors (Figure 1; Paganoni et al. 2021).

The significance of this finding is that it allows proximity sensors to be re-used within a defined battery life or within a lambing season, thereby reducing the cost of devices per animal. Flock demographics did not affect sensor success and hence accuracy. There was no convincing evidence that dam age, lamb birth type, paddock size, mob size or stocking rate, or lamb age up to 80-days influenced the success of the sensors. Sohi et al. (2017) reported a lower frequency of ewe-lamb interactions for three-week-old lambs than for one and two-week-old lambs, which was consistent with previous reports that a dam’s interactions in her lambs declines with increasing age of the lamb (Dwyer 2014). If these changes in ewe-lamb interactions exist, they were of little practical significance, as maximum success was achieved within 24 hours, regardless of lamb age up 80 days. Only one site had lambs older than 100 days, and sensor success declined to 48% for lambs older than 125 days, suggesting that older lambs may have begun self-weaning, decreasing the time spent with their dam, and therefore did not return accurate results. This potential decline in success of proximity sensors in older lambs does not limit their application commercially as lambs are typically weaned before 100 days old.
The cost to identify the dam pedigree of a lamb using proximity sensors was estimated at between $9 and $11, per lamb (Table 1).
Pedigree method | No. of farms | Cost per lamb | Comments |
Pedigree Matchmaker | 2 | $3-4 | Changes the sheep’s behaviour. Difficult to set up and learn how to use initially. Can be difficult to capture data. Birth weights not captured. |
Mothering up in yards | 1 | $6-7 | Low-tech method to capture pedigree. Birth weights not captured. |
Proximity sensors | 40 | $9-11 | No change in sheep behaviour required. The largest component of cost was to analyse the data. Birth weights not captured. |
Mothering up at birth | 2 | $14-18 | Most time intensive pedigree method. Captures birth weight, birth date and birth type of lambs. |
DNA analysis | 1 | $25-30 | Captures more genetic data, requires the ewes and rams to be analysed. Cost to process sample is high. Birth weights not captured. |
The largest cost was the data analysis fee (Table 2), so improving the speed and efficiency of processing data from proximity sensors to generate pedigree reports should be a priority.
Scenario | Cost |
200 ewes in one mob, $2.50 analysis | $11.10 |
400 ewes in one mob, $2.50 analysis | $10.70 |
400 ewes in two mobs, $2.50 analysis | $9.30 |
400 ewes in two mobs, $2.00 analysis | $8.40 |
400 ewes in two mobs, $1.50 analysis | $7.50 |
Current pricing indicates that proximity sensors are more expensive than Pedigree Matchmaker and mothering up in the yard, however these methods can involve significant labour costs and skills to set up and/or complete, and hence have been poorly adopted by industry. Furthermore, it can be estimated via comparisons with the sensor data that the accuracy in establishing dam pedigree was lower for farms using Pedigree Matchmaker or mothering up in the yards. If confirmed across a larger number of flocks, the lower reliability of these methods would reduce the accuracy of selection and rate of genetic gain that could be achieved (van der Werf 2010). Mothering up at birth and DNA testing cost significantly more than proximity sensors, but these methods also have other values, such as birth weight information that can be collected when mothering up, and extra genomic information that can be analysed via DNA. Other advantages in using proximity sensors, that are yet to be discovered, such as indicators of mothering ability, milk production and growth rates, are likely. The most appropriate pedigree method will likely depend on the objectives of individual producers, and their ability to provide the necessary labour requirements and resources. Overwhelmingly, using proximity sensors appears to be a promising alternative that may have other practical applications.
For all pedigree methods, errors are introduced through mis-recording sheep IDs, failing to measure all sheep (for example, failed blood cards, not sampling/applying collars to all sheep), mismothering at (or shortly after) birth, and working with novel technology. The accuracy of each method will be determined by the effort and success in reducing errors, and thereby improving the cost effectiveness of pedigree recording. The level of accuracy achieved commercially is likely to be lower than what we achieved in this study, as producers face challenges that could reduce accuracy. The most significant challenge is limited knowledge and expertise in the effective use of technology, at least initially. It is also possible that producers will fail to invest enough time in ensuring they accurately record information, and the necessary equipment (eID reader/laptop/other recording device). For adoption this technology must be straightforward, as initial failure could impact uptake.
This study provides convincing evidence that proximity sensors provide a rapid and accurate method of establishing maternal pedigree at relatively low cost, and the method is robust across a range of conditions experienced on commercial properties across southern Australia. Our study also provides a protocol for measurement of dam pedigree using proximity sensors. It is envisaged that private industry can now develop more cost effective sensor technologies with greater confidence. The development of these new products should focus on reducing the upfront and on-going costs of data analysis. It is inevitable that a range of lower cost products will be in the marketplace within a decade. This technology should enhance the recording of dam pedigree, and hence the rate of genetic gain across the sheep industry. In the longer term, the benefits from using sensor technologies for measurement of dam pedigree will be dwarfed using sensors for other applications, such as determining sire pedigree, behaviours, and as indicators of health and welfare, which will have much broader application across the sheep industry.
Conclusions
Proximity sensors provide a rapid, accurate and robust method for establishing dam pedigree that is cheaper than the existing methods of mothering lambs to ewes and blood sampling for DNA. Comparing the lamb and ewe with the first most interactions to the lamb and ewe with the second most interactions, at ratios above two, gives a confident dam result irrespective of flock demographics or paddock size.
References
Dodds KG, Tate ML, Sise JA (2005). Genetic evaluation using parentage information from genetic markers. J. Anim. Sci. 83: 2271-2279
Dwyer CM (2014). Maternal behaviour and lamb survival: From neuroendocrinology to practical application. Animal 8(1); 102-112.
Lee GJ, Atkins KD, Sladek MA (2009). Anim. Prod. Sci. 49: 624.
Lockwood A, Hancock S, Paganoni B, Macleay C, Kearney G, Sohi R and Thompson A (2018). Mob size of single-bearing or twin-bearing Merino ewes at lambing may not influence lamb survival at lambing when feed-on-offer is high. Animal 13: 1311-1318
McLaren CE (1997). Dry sheep equivalents for different classes of livestock. Department of Primary Industries, Victoria. ISSN 1329-8062.
Paganoni BL, Macleay CM, Scanlan V, Thompson AN (2021). Proximity sensors provide an accurate alternative for measuring maternal pedigree of lambs in Australian sheep flocks under commercial conditions. Anim. Prod. Sci. 61: 1951-1957. https://doi.org/10.1071/AN21190
Richards JS, Atkins KD (2007). Determining Pedigree by Association in Merino flocks. Proc. Assoc. Admt. Anim. Breed. Genet. 17: 403-406.
Richards J, Atkins K, Mortimer M, Semple S (2007). Pedigree assignment by electronic matching of lambs and dams. Trangie QPLUS Merinos. In ‘Proceedings of the Trangie QPLUS Open Day, Trangie Agricultural Research Centre, Trangie, Australia, 7 June 2007’. pp. 42–43.
Sohi R, Trompf J, Marriott H, Bervan A, Godoy BI, Weerasinghe M, Desai A, Jois M (2017). Determination of maternal pedigree and ewe-lamb spatial relationships by application of Bluetooth technology in extensive farming systems. J. Anim. Sci. 95; 5145-5150.
Van der Werf J (2010). Value of alternative methods to determine parentage. MLA Final Report B.BSC.0094.
DXA calibration at WAMMCO, Katanning
Steve Connaughton, Murdoch University Perth, WA
Author correspondence: s.connaughton@murdoch.edu.au
Introduction
Dual Energy X-ray Absorptiometry (DXA) is an imaging method shown to predict lamb carcass composition with a very high level of precision and accuracy. It uses two x-ray images produced at different photon energies, allowing for the differentiation of fat, lean muscle, and bone within the lamb carcass. There are five large abattoirs in Australia that have DXA installed and operating at chain speed, providing real-time carcass composition results. The most recent installation was at WAMMCO, Katanning, in southern Western Australia. This work focused on the calibration of the DXA and ability of the DXA to accurately predict lamb carcass composition.
Overview
In early August 2021, 201 lambs from the Meat and Livestock Australia (MLA) resource flock at the Department of Primary Industries and Regional Development’s (DPIRD) Katanning Research Facility were slaughtered at WAMMCO. These lambs are of a known and varied phenotypic and genotypic range. All lambs were placed through the DXA scanner, and 100 of these were selected to be CT scanned at Murdoch University, one to two days post-slaughter. CT scanning is the gold standard for determining carcass composition, allowing DXA predictions of composition to be directly compared to it and validated. The comparison between CT and DXA predictions of carcass composition was assessed, with a synthetic phantom block used to calibrate the WAMMCO DXA to the algorithm used at the original Bordertown DXA in South Australia.
Methods and materials
The WAMMCO facility processed 201 lambs, with a spread of Hot Carcass Weight (HCWT) from 12.8 to 30.9kg (mean 22.25kg) and GR tissue depth of 1mm to 32mm (mean 9.65mm). 100 of these lambs were selected to optimise the spread of phenotypes (Table 1) and genotypes and sent to be CT scanned at Murdoch University. The spread of HCWT and GR measurements for all lambs can be seen in Figure 1.
HCWT (kg) | GR (mm) | |||||||
Mean | SD | Min | Max | Mean | SD | Min | Max | |
CT Group | 22.35 | 2.804 | 12.8 | 29.4 | 9.63 | 4.584 | 1 | 21 |
Non-CT Group | 22.16 | 2.958 | 13.9 | 30.9 | 9.67 | 5.158 | 1 | 32 |
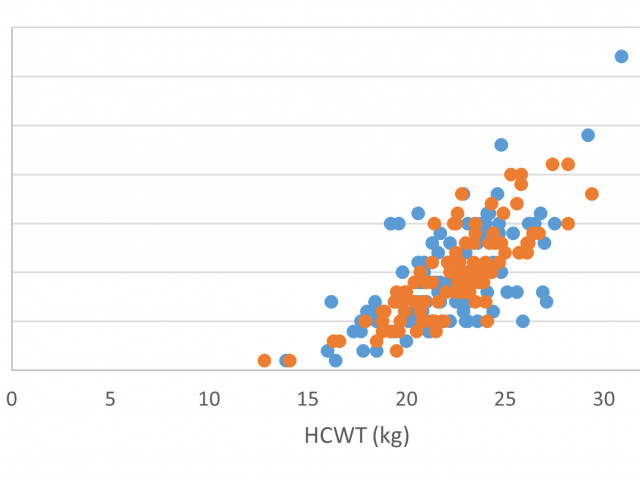
Fat score and GR predictions versus CT predictions
Fat score by palpation and GR tissue depth by GR knife were measured and compared to the CT fat percentage. Palpated fat score directly predicted CT fat percentage with poor precision, with R2=0.27 and RMSE=3.87% (Figure 2).
GR measurement using a GR knife directly predicted CT fat percentage better than palpated fat score, with R2=0.59 and RMSE=2.88% (Figure 3). The addition of HCWT with GR in predicting CT fat percentage was not significant.
Calibrated DXA predictions versus CT predictions
A mix of plastic ‘phantoms’ was scanned during this calibration experiment, which allowed us to correct the WAMMCO DXA in line with the original calibration site in Australia. This correction gave excellent results, as has been done at all previous DXA sites around Australia.
The calibrated DXA predictions of CT fat, lean and bone percentages are comparable to the CT determinations of compositions (Tables 2 and 3).
CT Fat % | CT Lean % | CT Bone % | ||||||
Mean ± SD | Min | Max | Mean ± SD | Min | Max | Mean ± SD | Min | Max |
22.55 ± 4.38 | 11.63 | 33.09 | 58.56 ± 3.23 | 51.61 | 68.71 | 18.89 ± 1.89 | 15.24 | 23.22 |
DXA Fat % | DXA Lean % | DXA Bone % | ||||||
Mean ± SD | Min | Max | Mean ± SD | Min | Max | Mean ± SD | Min | Max |
22.77 ± 4.06 | 11.77 | 30.82 | 58.94 ± 2.89 | 53.22 | 66.76 | 18.28 ± 1.17 | 15.96 | 21.46 |
There is a small slope and bias evident in the fat, lean and bone percentage predictions, however the precision demonstrated here is very high (Table 4) with R2=0.89 and RMSE=1.46% for DXA fat percentage predictions and R2=0.78 and RMSE=1.52% for DXA lean percentage predictions. This is comparable to other DXA sites around Australia. The precision and accuracy of the fat percentage predictions can be visualised in Figure 4.
DXA Fat % | DXA Lean % | DXA Bone % | |
R2 | 0.89 | 0.78 | 0.46 |
RMSE | 1.46% | 1.52% | 1.39% |
Slope | 1.02 | 0.99 | 1.09 |
Bias | 0.22 | 0.38 | -0.60 |
Conclusion
The DXA system at WAMMCO was successfully calibrated against the other systems in Australia. It is now producing numbers with a high level of precision and accuracy. Importantly, this experiment has highlighted the enormous improvement that DXA has made in predictions of carcass composition, particularly fatness, compared to the current Australian standard of GR measurement or palpated fat score.
The feedback producers will be able to receive about their lamb mob from DXA is far richer and more precise than the information currently available and will allow producers to make effective decisions about future genetic selection and farm management decisions. This may become important when DXA composition values become a trait that can be traded upon by the sheep meat industry in future.
New WA NLIS Sheep and Goat Advisory Group established
Beth Green, DPIRD Bunbury, WA
Author correspondence: beth.green@dpird.wa.gov.au
On Thursday, 11 November 2021, 31 people attended the first meeting of the Western Australian National Livestock Identification Scheme (NLIS) Sheep and Goat Advisory Group at the Department of Primary Industries and Regional Development (DPIRD), in South Perth. Attendees represented every sector of the sheep and goat industries, WA farming organisations, Pastoralists and Graziers Association and WAFarmers, and WA members of national peak councils, Sheep Producers Australia and Wool Producers Australia.
The group was convened because of growing national discussions to enhance traceability of all livestock, to better support the long-term health and prosperity of our industry and access to markets. This involves addressing recommendations put to the National Biosecurity Committee (NBC) by industry and government, through SAFEMEAT*, to:
- Invest in a movement database for all Foot and Mouth Disease-susceptible (cloven-hooved) livestock species.
- Mandate individual electronic identification (eID) for all livestock, beginning with sheep and goats, and
- Ensure equitable cost-sharing for the system.
The Advisory Group’s Chair, Beth Green, Manager of Livestock Identification and Traceability in WA for DPIRD, opened with the purpose of the group:
- To review, monitor and identify areas for development, and assess options for improvement in traceability across the sheep and goat supply line for all commercial and non-commercial operators.
- To provide engagement and discussion with DPIRD for the path forward in WA, and
- To discuss the strategic and operational issues relating to enhanced identification and movement recording to support our markets and disease response.
Following peak industry support through SAFEMEAT* for the introduction of mandatory eID for sheep and goats in Australia, NBC support had also been reached, but any decision on when and how to implement eID has not yet been made. Further work on developing cost estimates and funding agreements are required before a national decision is considered by agriculture ministers.
The ‘how’ in WA can be influenced by this Advisory Group. WA industry experience and leadership will be required to guide the development of future planning for any transition to eID. Research into the most appropriate infrastructure, policy discussion and provision of ongoing technical support and training, underpinned by adequate funding, will be needed to manage the transition well.
DPIRD commissioned a consultancy by ACIL Allen to review the lessons learnt from Victoria’s move to mandatory eID in sheep and goats. A second report is underway to investigate the infrastructure needed throughout the supply chain to generate a realistic cost estimate – from tagging on property to slaughter or loading onto ships. These reports will be shared with the Advisory Group and allow WA to learn from the Victorian experience and develop funding proposals based on a credible and informed design.
Any move to eID must maintain or improve flow rate of stock, have technical capability for at least 99% traceability, and provide WA with an accurate understanding of costs to ensure adequate implementation funding, with minimal disruption.
Moving towards eID will be a significant part of the Advisory Group’s agenda. However, tag type is not the whole system and improvements in the existing system can be identified and resolved now, to contribute to enhanced traceability.
Producer and WA Farmers representative, David Slade, supported the need for enhanced traceability stating, “Industry needs to be accountable to our markets and maintain credibility of production and traceability claims for food safety.”
WA Chief Veterinary Officer, Michelle Rodan, explained that traceability through the sheep and goat supply chains is critical. Australia’s ability to manage disease is dependent on how rapidly it can be traced, which is critical for providing assurances to our markets. If our traceability data is credible, WA has the potential to zone off from the rest of Australia in the event of a disease outbreak.
In WA, the Biosecurity and Agricultural Management (Identification and Movement of Stock and Apiaries) Regulations 2013 (BAM [IMSA] regulations) outline the requirements for:
- Owner registration
- Property identification
- Stock identification
- Waybills, and
- Recording stock movements on the National Livestock Identification System (NLIS) database.
These components work together to create a system of collecting traceability data. Adherence to the regulations at all points of stock movement ensures the data is accessible and accurate, in preparation for a future emergency response. Locating missing data or responding in its absence will slow a response, which could exacerbate the losses and down time. With the impact of Covid-19, this has become acutely familiar to everyone.
The backbone of traceability through the NLIS is the requirement for the destination owner/receiver to record the movement of stock to their property on the NLIS database. Many producers are not aware of the NLIS database, nor their responsibilities to record livestock movements onto their Property Identification Code (PIC), even if they own both PICs (unless purchased from a saleyard, in which case the saleyard will do it for them).
At the meeting, discussion from participants of each sector focused on identifying the current friction points in the industry, how to improve the flow rate of stock within each location, and how to improve the accuracy of the data.
The following are issues for all of industry:
- National Vendor Declaration (NVD)/waybills are regularly incomplete or illegible at all destinations.
- Using the electronic NVD/waybill helps with legibility and completeness.
- The use of electronic NVD/waybill has suffered problems with destination addresses, correct destination PICs, accurate tallies, and the need to sign three times, and some transport operators are not yet comfortable with them.
- The NLIS database is difficult to use for people who do not use it regularly.
- More extension is required for both NVD/waybills and NLIS database use.
- Missing or worn/unreadable tags required for tagging at export depots/feedlots and saleyards.
- Expired brand registrations and consequently Livestock Production Assurance (LPA) accreditation, and old NVD versions are time-consuming to follow up on, and
- Counting discrepancies of animal numbers between producer, NVD/waybill, saleyards, transporters, and processors.
This initial meeting was more of a workshop, as it established the baseline operating processes and hindrances, and established the current national position. Future meetings will be structured to cover prioritising and improving current components, planning, and taking a strategic view on how traceability fits within the bigger production picture.
The next meeting will be in late February/early March 2022. For further information, please contact Jemma Thomas at Katanning DPIRD on M: 0459 850 569 or at E: jemma.thomas@dpird.wa.gov.au; or contact your industry sector representative:
Industry sector representatives |
|
Sheep Producers Australia | Liz Jackson, Bindi Murray |
Wool Producers Australia | Steve McGuire |
Pastoralists and Graziers Association | Digby Stretch, Ian Randles |
WAFarmers | David Slade, Jess Wallace |
Dairy Goats Society of Australia (WA) | Dave Robinson |
WA Goat Meat Industry Council | Trevor Bunce |
Grower Group Alliance | Alison Lacey |
Stud breeder | Allan Hobley |
WAMIA, Muchea Saleyards | Steve Wainewright, Liam Birken |
Katanning Saleyards | Rod Bushell |
Processor sector | Cam Ferris (Hillside Processing) |
Export sector | Harold Sealy |
Feedlot sector | Alan Garstone, Hannah Matthews |
Livestock Agents | Don Morgan (AWN) |
| Grant Lupton (Nutrien) |
| Mike Curnick (Elders) |
| Gerald Wetherell (West Coast Wool and Livestock) |
Transporters | Jan Cooper (ALRTA(WA)) |
SAFEMEAT industry members (peak councils) | Government members | Observers |
Australian Dairy Farmers (ADF) | State Food Authority Representative | Animal Health Australia (AHA) |
Australian Live Export Corporation (ALEC) | Agriculture Victoria | Australian Livestock and Property Agents Association (ALPA) |
Australian Lot Feeders’ Association (ALFA) | Australian Department of Agriculture, Water and the Environment | Australian Livestock Markets Association (ALMA) |
Australian Meat Industry Council (AMIC) | Department of Primary Industries, Parks, Water and Environment, TAS | Australian Livestock and Rural Transporters’ Association (ALRTA) |
Australian Pork Limited (APL) | Department of Primary Industries and Regional Development, WA | Australian Meat Processor Corporation (AMPC) |
Cattle Council of Australia (CCA) | Department of Industry, Tourism and Trade, NT | Australian Pesticides and Veterinary Medicines Authority (APVMA) |
Goat Industry Council of Australia (GICA) | NSW Department of Primary Industries | Australian Renderers’ Association |
Sheep Producers Australia (SPA) | Primary Industries and Regions SA | Dairy Australia |
| QLD Department of Agriculture and Fisheries | Integrity Systems Company (ISC) |
|
| Kangaroo Industry Association of Australia |
|
| Meat & Livestock Australia (MLA) |
|
| Stock Feed Manufacturers Council of Australia |
|
| Wool Producers Australia (WPA) |
Optional sheep ear marking comes into effect from 1 January 2022
From 1 January 2022, earmarking of sheep and earmarking and/or branding of cattle (including buffalo) will be optional.
Following consultation undertaken by the Department of Primary Industries and Regional Development (DPIRD) in 2020, changes have been made to the Biosecurity and Agriculture Management (Identification and Movement of Stock and Apiaries) Regulations 2013 for the identification of sheep and cattle (including buffalo).
Sheep identification requirements:
- Earmarking of sheep is optional from 1 January 2022. This means that it is up to each owner to choose whether to earmark their sheep.
- Some owners may wish to reduce the costs, labour, time and physical impacts on their stock by ceasing to earmark, while others may retain earmarking as an additional form of identification, such as for farm management purposes.
- Owners who continue to earmark will be required to use the registered earmark allocated to them, as listed on their PIC card.
- NLIS tagging on property of birth, with an accredited year of birth-coloured NLIS visual or electronic tag, remains mandatory.
- NLIS tagging with an additional pink tag, prior to leaving any owner’s property, remains mandatory.
For further information on the requirements for identification and traceability of sheep, please visit agric.wa.gov.au/livestock-biosecurity/livestock-identification-and-movement-sheep